Can Nvidia Maintain Its Position in the AI Chip Arms Race?
Follow me on Moomoo to stay informed and connected!
1. Due to the strong demand for AI computing power, major tech giants, including Nvidia, have engaged in an "arms race" in AI chip development.
Several key players in the technology industry have made significant advancements in AI chip development.
Tesla has successfully initiated mass production of its proprietary chip, named Dojo.
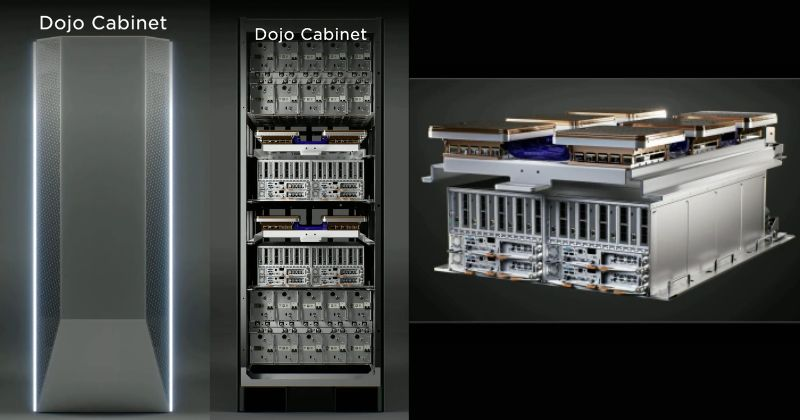
At the annual Google Cloud Next event, Google unveiled the fifth-generation custom tensor processing unit (TPU) chip, TPU v5e, specifically designed for large-scale model training and inference.
Amazon AWS has integrated its in-house Trainium and Inferentia chips for training and inference purposes.
Microsoft is also in the process of planning the release of its self-developed Athena chip.
AMD has introduced the AI chip MI300X , which boasts a memory capacity of 192GB and supports AI inference capabilities. Additionally, Intel has secured substantial orders worth $1 billion for its AI chips, including the Gaudi2 and Gaudi3 chips.
Given the fierce competition and advancements made by these companies, it raises the question of whether Nvidia's leading position in the AI chip market remains unchallenged.
2. NVIDIA GH200 Grace Hopper Chip Leading Competitors
Based on the test data, NVIDIA continues to maintain its leading position in terms of chip performance across various metrics. On August 8, 2023, Jensen Huang, the CEO of NVIDIA, introduced the next-generation GH200 Grace Hopper platform at SIGGRAPH 2023. This platform is specifically designed for generative AI and is capable of running almost any large-scale language model with exceptional performance in inference tasks. On September 11, the NVIDIA GH200 Grace Hopper superchip made its debut in the MLPerf industry benchmark test, demonstrating significant improvement. It achieved a 17% performance boost compared to the H100 GPU. These results indicate that NVIDIA continues to lead in both training and inference, which are crucial aspects of GPU applications for AI models.
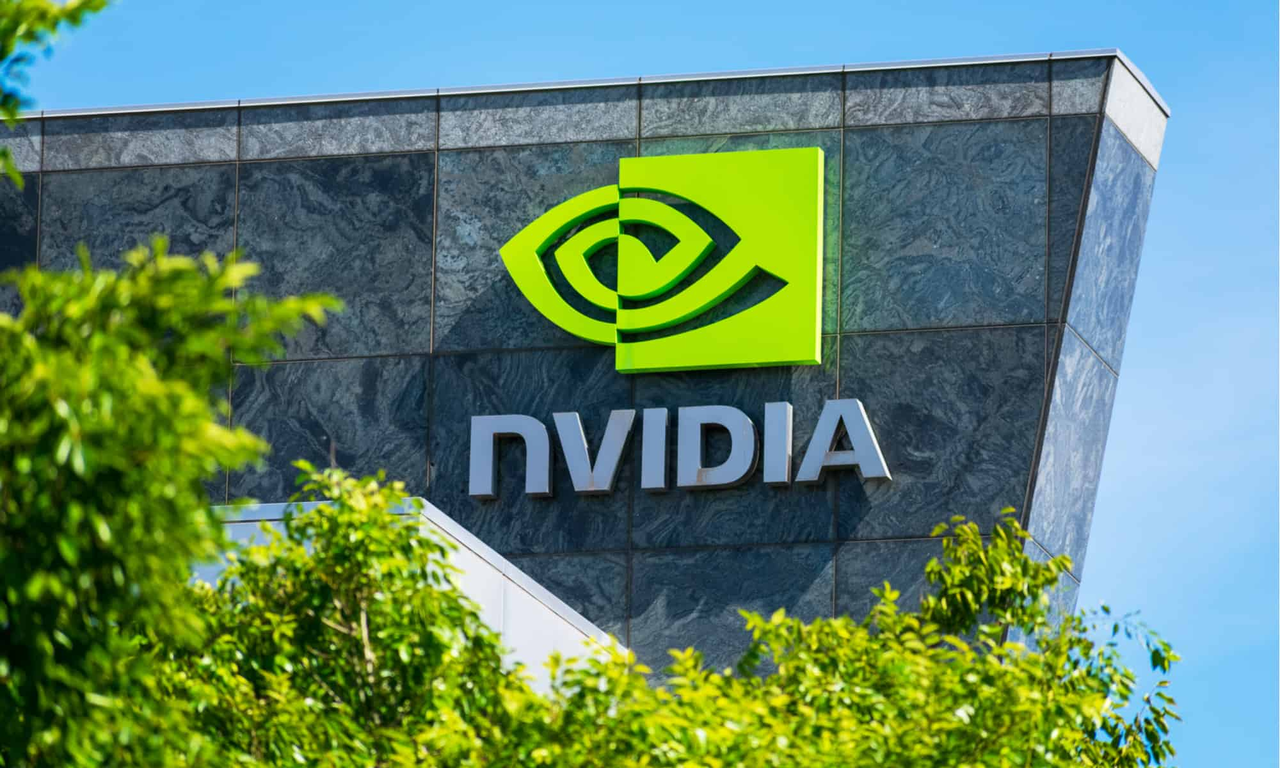
Despite the intensifying competition in the AI chip market, NVIDIA's strong array of technologies and comprehensive product portfolio allow them to maintain a competitive advantage. They have consistently delivered powerful solutions that cater to the demands of AI applications. The advancements made by other companies mentioned earlier are noteworthy, but NVIDIA's established position, track record, and continuous innovation contribute to their continued dominance in the AI chip market.
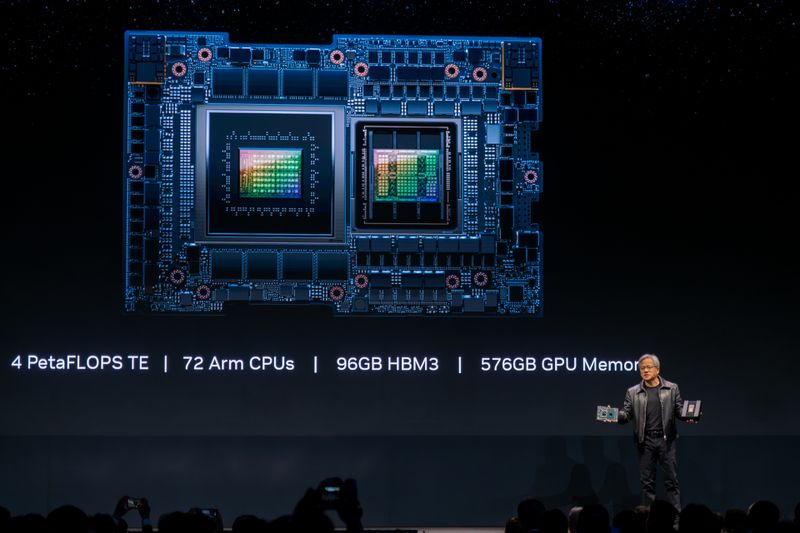
3. NVIDIA's advantage lies not only in hardware but also in the CUDA ecosystem.
In recent years, Google's TPU and Tesla's Dojo have demonstrated impressive computing capabilities. It is possible that in the future, these technologies could catch up to and even surpass NVIDIA in terms of raw computing power. However, NVIDIA's competitive advantage extends beyond hardware. The company has successfully developed a robust ecosystem centered around CUDA, which serves as its strongest barrier.
CUDA is a parallel computing platform and programming model that enables developers to fully harness the computational power of NVIDIA GPUs. Presently, there are 15,000 startups built on the NVIDIA CUDA platform, and 40,000 large enterprises worldwide are utilizing CUDA for accelerated computing. In contrast, AMD's ROCm ecosystem, which they have been diligently working on, pales in comparison.
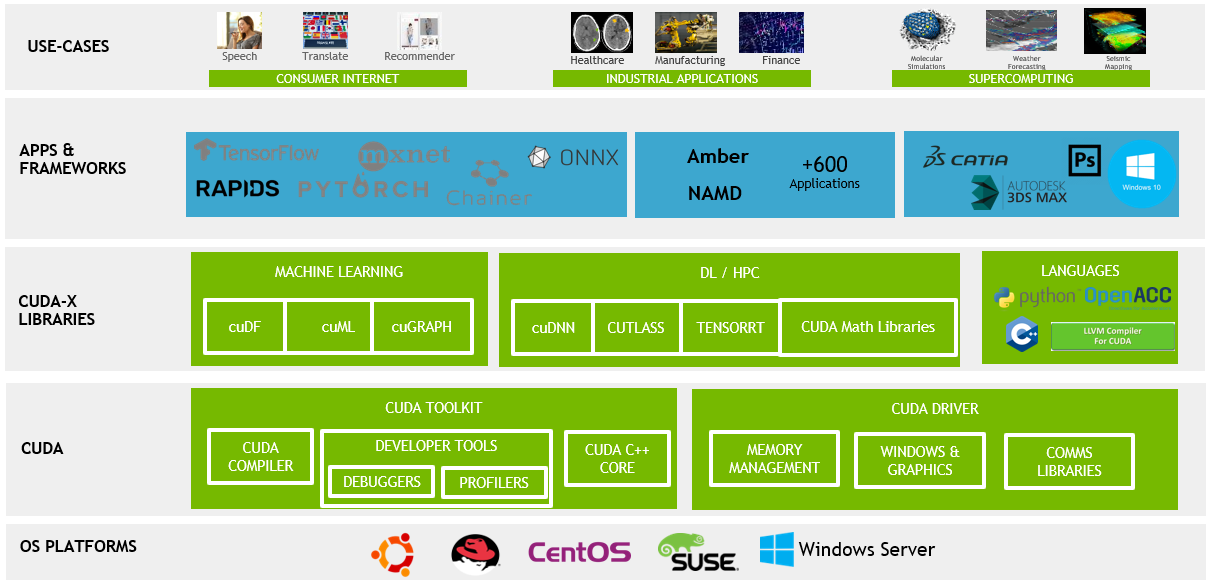
Regarding the CUDA ecosystem, Pete Warden, a researcher from Stanford University, has highlighted several reasons for NVIDIA's dominant position. NVIDIA's GPUs are the most efficient choice for artificial intelligence development, offering greater convenience and time savings compared to alternative options. Moreover, NVIDIA's CUDA ecosystem is more mature, providing abundant resources, support, and excellent integration with major frameworks such as PyTorch and TensorFlow.
There are two key factors contributing to NVIDIA's stronghold in the field:
High dependency : Exceptional AI talent is scarce, and the cost of hiring and retaining researchers is substantial. Given researchers' preference for familiar tools, companies often prioritize purchasing NVIDIA platforms when acquiring hardware. Using NVIDIA GPUs is more convenient and time-saving compared to alternatives such as AMD OpenCL cards, Google TPUs, Cerebras systems, or other hardware options. This improves efficiency and meets the needs of researchers.
High irreplaceability: Researchers typically iterate on existing models within controlled training times. NVIDIA's GPUs, which are consistently updated, offer faster training speeds, and existing code can seamlessly run on the latest hardware. Switching to hardware from other companies would require restructuring the code, which is a time-consuming and labor-intensive process. While NVIDIA's competitors may theoretically offer lower latency, NVIDIA's investments and accumulation in software stacks make this advantage mostly a fantasy at present.
3. Competition in the self-developed chip market is intense. Can NVIDIA maintain its current position?
The major technology giants are encountering challenges such as shortages in GPU supply and rapidly escalating costs, compelling them to explore the development of their own chips. However, at present, these companies' self-developed chips can only be utilized internally and are not widely available for promotion. Moreover, due to the preference of downstream customers' AI engineers for NVIDIA GPUs, even if these tech giants have engineered their own chips, they still need to procure NVIDIA's chips to meet their customers' demands.
For instance, Tesla's Dojo chip is an exclusive chip designed specifically to cater to their own requirements. It distinguishes itself from NVIDIA's general-purpose chips and cannot be fully substituted for them. Consequently, licensing Dojo for third-party use poses a challenge. While it is relatively straightforward for small and medium-sized companies to hire individuals proficient in NVIDIA CUDA programming, finding personnel skilled in Dojo or other programming languages proves to be difficult. In other words, if a small or medium-sized company were to adopt Dojo, it would incur significant learning costs and encounter challenges in recruitment. Furthermore, given that Dojo was extensively customized from its inception, it is nearly impossible for companies outside of Tesla to replace NVIDIA's GPUs.
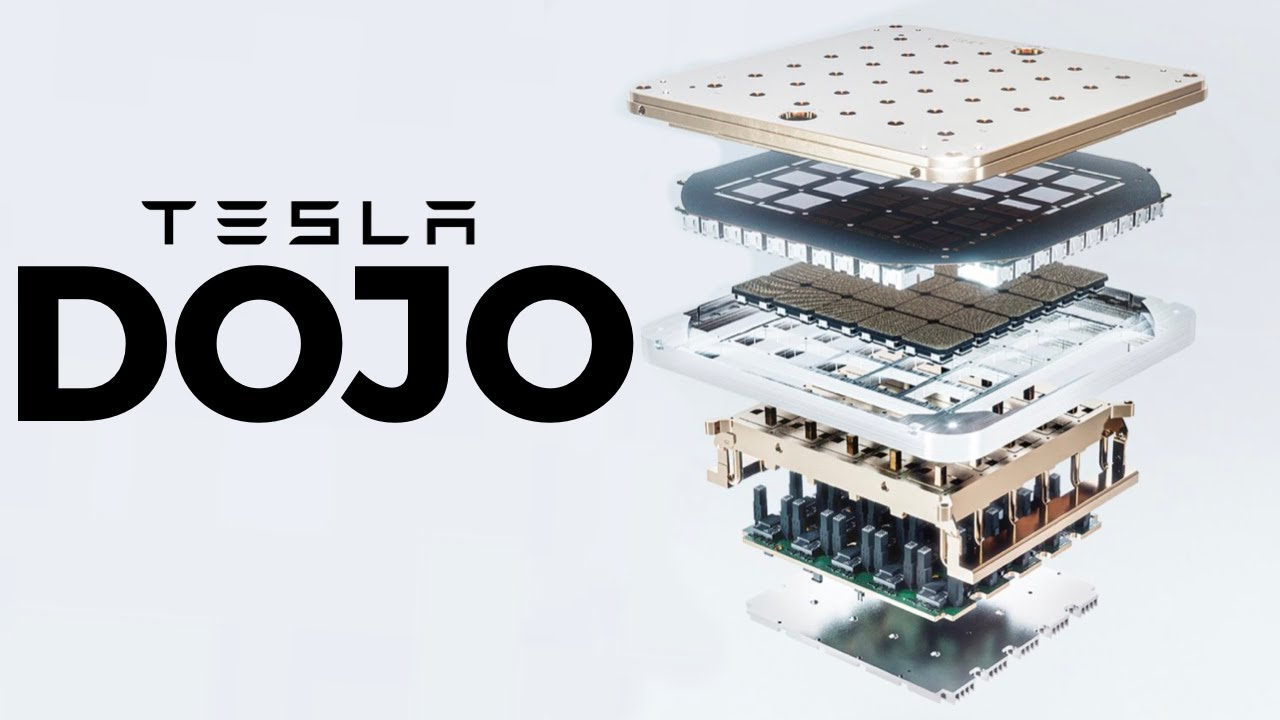
Google also confronts similar issues. Initially designed for internal purposes, Google's TPU has evolved into the TPU v5e, capable of efficiently scaling various AI workloads, including training, fine-tuning, and inference. TPU provides multiple functionalities and accelerates workloads on AI frameworks like PyTorch, JAX, and TensorFlow. However, despite the introduction of powerful fifth-generation TPU chips, Google continues to offer NVIDIA chips on their cloud platform. This underscores the harsh reality that Google must confront: many AI engineers prefer utilizing NVIDIA GPUs.
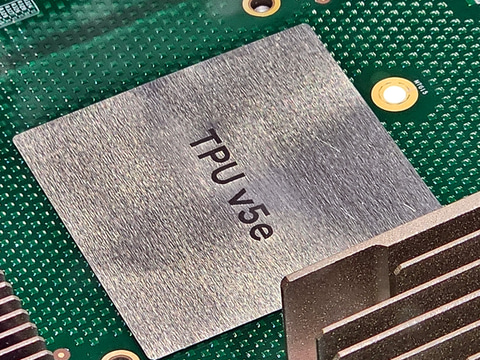
4. Conclusion
Nvidia maintains a competitive advantage in the AI chip market due to its robust technological capabilities, diverse product portfolio, and strong partnerships.
One of Nvidia's significant competitive advantages lies in its comprehensive ecosystem, which goes beyond hardware. The CUDA parallel computing platform and programming model offered by Nvidia provide developers with more efficient options for AI development. This platform is supported by a mature ecosystem that offers abundant resources and support.
Although Tesla's Dojo and Google's TPU are powerful chips, they cannot completely replace Nvidia's position in the market.
As the field of artificial intelligence continues to rapidly evolve, new architectures and dedicated chips may emerge to meet the growing demands. GPUs may not be the sole solution.
Disclaimer: Community is offered by Moomoo Technologies Inc. and is for educational purposes only.
Read more
Comment
Sign in to post a comment